AI in the assessment of Barrett's neoplasia
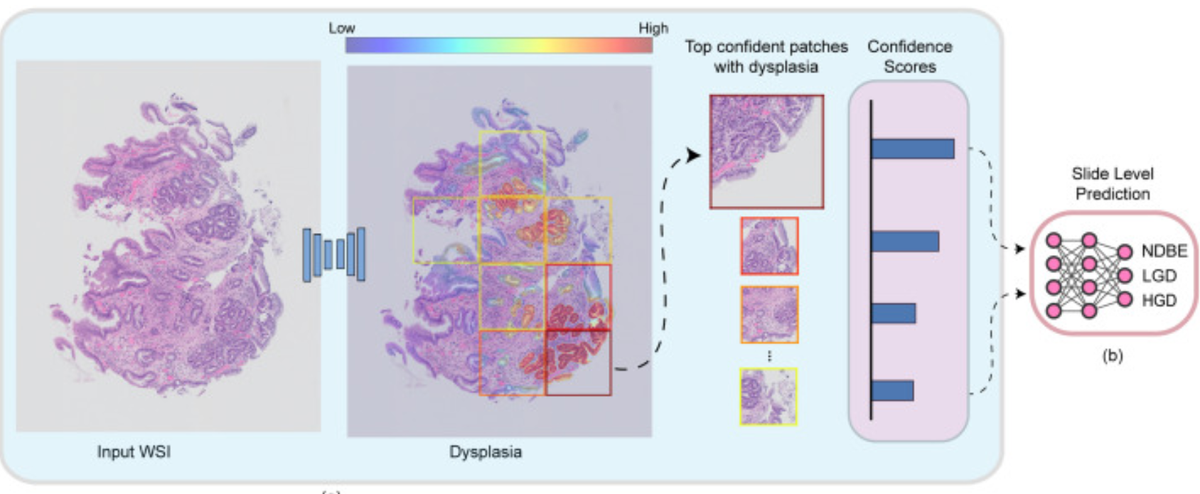
Accurate and timely identification of Barrett’s-related (BE) dysplasia is critical to providing the timely surveillance and treatment needed to prevent progression to esophageal adenocarcinoma. Unfortunately, significant observer variability in the assessment of BE-related dysplasia by pathologists continues to be a challenge. This report discusses the development and application of an artificial intelligence (AI) system for histopathological assessment of BE-related dysplasia. The AI system correctly graded 76.4% of the images, surpassing the performance of almost all of the participating pathologists. However, the misclassification of almost a quarter of the cases illustrates the challenges inherent in approaches that are based solely on histology. The real-world behavior of such systems is still unknown and needs further investigation. [Edit: Others have also recently explored this topic (Meinikheim, et al. 2024), suggesting that now is the time to examine approaches that combine machine learning and human expertise (what a concept!) (De Groof, 2024)]
Deep learning for histopathological assessment of esophageal adenocarcinoma precursor lesions.
Botros M, de Boer OJ, Cardenas B, Bekkers EJ, Jansen M, van der Wel MJ, Sánchez CI, Meijer SL.
Abstract
Histopathological assessment of esophageal biopsies is a key part in the management of patients with Barrett's esophagus (BE) but prone to observer variability and reliable diagnostic methods are needed. Artificial intelligence (AI) is emerging as a powerful tool for aided diagnosis but often relies on abstract test and validation sets while real world behavior is unknown. In this study, we developed a two-stage AI system for histopathological assessment of BE-related dysplasia using deep learning to enhance the efficiency and accuracy of the pathology workflow. The AI system was developed and trained on 290 whole slide images (WSIs) that were annotated at glandular and tissue level. The system was designed to identify individual glands, grade dysplasia, and assign a WSI-level diagnosis. The proposed method is evaluated by comparing the performance of our AI system to a large international and heterogeneous group of 55 GI pathologists assessing 55 digitized biopsies spanning the complete spectrum of BE-related dysplasia. The AI system correctly graded 76.4% of the WSIs, surpassing the performance of 53 out of the 55 participating pathologists. Furthermore, the ROC analysis showed that the system's ability to predict the absence (non-dysplastic BE) versus the presence of any dysplasia with an AUC of 0.94 and a sensitivity of 0.92 at a specificity of 0.94. These findings demonstrate that this AI system has the potential to assist pathologists in assessment of BE-related dysplasia. The system's outputs could provide a reliable and consistent secondary diagnosis in challenging cases or be used for triaging low-risk non-dysplastic biopsies, thereby reducing the workload of pathologists, and increasing throughput.